During the past four months, Magnum began its adventure into the Python world. Not just with some autogenerated bindings and not just with some autogenerated Sphinx docs — that simply wouldn’t be Magnum enough. Brace yourselves, this article will show you everything.
The new Magnum Python bindings, while still labeled experimental, already give you a package usable in real workflows — a NumPy-compatible container library, graphics-oriented math classes and functions, OpenGL buffer, mesh, shader and texture APIs, image and mesh data import and a SDL / GLFW application class with key and mouse events. Head over to the installation documentation to get it yourself; if you are on ArchLinux or use Homebrew, packages are already there, waiting for you:
brew tap mosra/magnum brew install --HEAD corrade magnum magnum-plugins magnum-bindings
And of course it has all goodies you’d expect from a “Python-native” library — full slicing support, errors reported through Python exceptions instead of return codes (or hard asserts) and properties instead of setters/getters where it makes sense. To get you a quick overview of how it looks and how is it used, the first few examples are ported to it:
Enter pybind11
I discovered pybind11 by a lucky accident in early 2018 and immediately had to try it. Learning the basics and exposing some minimal matrix/vector math took me about two hours. It was an extreme fun and I have to thank all pybind11 developers for making it so straightforward to use.
py::class_<Vector3>(m, "Vector3") .def_static("x_axis", &Vector3::xAxis, py::arg("length") = 1.0f) .def_static("y_axis", &Vector3::yAxis, py::arg("length") = 1.0f) .def_static("z_axis", &Vector3::zAxis, py::arg("length") = 1.0f) .def(py::init<Float, Float, Float>()) .def(py::init<Float>()) .def(py::self == py::self) .def(py::self != py::self) .def("is_zero", &Vector3::isZero) .def("is_normalized", &Vector3::isNormalized) .def(py::self += py::self) .def(py::self + py::self) .def(py::self *= Float{}) .def(py::self * Float{}) .def(py::self *= py::self)
That’s what it took to bind a vector class.
However, different things took a priority and so the prototype got shelved until it got revived again this year. But I learned one main thing — even just the math classes alone were something so useful that I kept the built Python module around and used it from time to time as an enhanced calculator. Now, with the magnum.math module being almost complete, it’s an everyday tool I use for quick calculations. Feel free to do the same.
>>> from magnum import * >>> Matrix3.rotation(Deg(45)) Matrix(0.707107, -0.707107, 0, 0.707107, 0.707107, 0, 0, 0, 1)
Quick, where are the minus signs in a 2D rotation matrix?
What Python APIs (and docs) could learn from C++
Every time someone told me they’re using numpy for “doing math quickly in Python”, I assumed it’s the reasonable thing to do — until I actually tried to use it. I get that my use case of 4×4 matrices at most might not align well with NumPy’s goals, but the problem is, as far as I know, there’s no full-featured math library for Python that would give me the whole package1 including Quaternions or 2D/3D transformation matrices.
As an excercise, for usability comparison I tried to express the rotation matrix shown in the box above in SciPy / NumPy. It took me a good half an hour of staring at the docs of scipy.spatial.transform.Rotation until I ultimately decided it’s not worth my time. The overarching problem I have with all those APIs is that it’s not clear at all what types I’m expected to feed to them and provided example code looks like I’m supposed to do half of the calculations myself anyway.
>>> from scipy.spatial.transform import Rotation as R >>> r = R.from_quat([0, 0, np.sin(np.pi/4), np.cos(np.pi/4)])
Rotation.from_quat(quat, normalized=False)
Parameters:
- quat: array_like, shape (N, 4) or (4,)
- Each row is a (possibly non-unit norm) quaternion in scalar-last (x, y, z, w) format.
Type information in the SciPy documentation is vague at best. Also, I’d like something that would make the quaternion for me, as well.
To avoid the type confusion, with Magnum Python bindings I decided to use
strong types where possible — so instead of a single dynamic matrix / vector
type akin to numpy.ndarray, there’s a clear distinction between matrices
and vectors of different sizes. So then if you do Matrix4x3() @ Matrix2x4()
,
docs of Matrix4x3.__matmul__() will tell you the result is Matrix2x3.
For NumPy itself, there’s a proposal for improved type annotations at
numpy/numpy#7370 which would help a lot, but the documentation tools
have to make use of that. More on that below.
One little thing with big impact of the C++ API is strongly-typed angles. You no longer need to remember that trig functions use radians internally but HSV colors or OpenAL juggles with degrees instead — simply use whatever you please. So Python got the Deg and Rad as well. Python doesn’t have any user-defined literals (and I’m not aware of any proposals to add it), however there’s a way to make Python recognize them. I’m not yet sure if this amount of magic is wise to apply, but I might try it out once.
- 1.
- ^ As /u/NihonNukite pointed out on Reddit, there’s Pyrr that provides the above missing functionality, fully integrated with numpy. The only potential downside is that it’s all pure Python, not optimized native code.
Hard things are suddenly easy if you use a different language
>>> a = Vector4(1.5, 0.3, -1.0, 1.0) >>> b = Vector4(7.2, 2.3, 1.1, 0.0) >>> a.wxy = b.xwz >>> a Vector(0, 1.1, -1, 7.2)
If you ever used GLSL or any other shader language, you probably fell in love with vector swizzles right at the moment you saw them … and then became sad after a realization that such APIs are practically impossible2 to have in C++. Swizzle operations are nevertheless useful and assigning each component separately would be a pain, so Magnum provides Math::gather() and Math::scatter() that allow you to express the above:
a = Math::scatter<'w', 'x', 'y'>(a, Math::gather<'x', 'w', 'z'>(b));
Verbose3 but practically possible. Point is, however, that the above is
implementable very easily in Python using __getattr__()
and
__setattr__()
… and a ton of error checking on top.
- 2.
- ^ GLM does have those, if you enable
GLM_FORCE_SWIZZLE
, but doing so adds three seconds4 to compilation time of each file that includes GLM headers. I’d say that makes swizzles possible in theory but such overhead makes them practically useless. - 3.
- ^ Math functions are functions and so do not mutate their arguments,
that’s why the final self-assignment. It would of course be better to be
able to write
Math::gather<"wxy">(b)
or at leastMath::gather<'wxy'>(b)
but C++ insists on the first being impossible and the second being unportable. And creating a user-defined literal just to specify a swizzle seems excessive. - 4.
- ^ I did a couple of benchmarks for a yet-to-be-published article comparing math library implementations, and this was a shocker. The only other library that could come close was Boost.Geometry, with two seconds per file.
… but on the contrary, C++ has it easier with overloads
I was very delighted upon discovering that pybind11 supports function overloads
just like that — if you bind more than one function of the same name, it’ll
take a typeless (*args, **kwargs)
and dispatch to a correct overload
based on argument types. It’s probably not blazingly fast (and in some cases
you could probably beat its speed by doing the dispatch youself), but it’s
there and much better than having to invent new names for overloaded functions
(and constructors!). With the new typing
module, it’s possible to achieve a similar thing in pure Python using the
@overload decorator — though only for documentation
purposes, you’re still responsible to implement the type dispatch yourself. In
case of math.dot() implemented in pure Python, this could look like
this:
@overload def dot(a: Quaternion, b: Quaternion) -> float: ... @overload def dot(a: Vector2, b: Vector2) -> float: ... def dot(a, b): # actual implementation
What was actually hard though, was the following, looking completely ordinary to a C++ programmer:
>>> a = Matrix3.translation((4.0, 2.0)) >>> a Matrix(1, 0, 4, 0, 1, 2, 0, 0, 1) >>> a.translation = Vector2(5.0, 3.0) >>> a Matrix(1, 0, 5, 0, 1, 3, 0, 0, 1)
Is the Python language police going to arrest me now?
While the case of Matrix3.scaling() vs. mat.scaling()
— where
the former returns a scaling Matrix3 and latter a scaling Vector3
out of a scaling matrix — was easier and could be done just via a dispatch
based on argument types (“if the first argument is an instance of Matrix3,
behave like the member function”), in case of Matrix3.translation() it’s
either a static method or an instance property. Ultimately I managed to solve
it by supplying a custom metaclass that does a correct dispatch when
encountering access to the translation
attribute.
But yeah, while almost anything is possible in Python, it could give a hand here — am I the first person ever that needs this functionality?
Zero-copy data transfer
One very important part of Python is the Buffer Protocol. It allows zero-copy sharing of arbitratily shaped data between C and Python — simple tightly-packed linear arrays, 2D matrices, or a green channel of a lower right quarter of an image flipped upside down. Having a full support for the buffer protocol was among the reasony why Containers::StridedArrayView went through a major redesign earlier this year. This strided array view is now exposed to Python as a containers.StridedArrayView1D (or MutableStridedArrayView1D, and their 2D, 3D and 4D variants) and thanks to the buffer protocol it can be seamlessly converted from and to numpy.array() (and Python’s own memoryview as well). Transitively that means you can unleash numpy-based Python algorithms directly on data coming out of ImageView2D.pixels() and have the modifications immediately reflected back in C++.
Because, again, having a specialized type with further restrictions makes the code easier to reason about, containers.ArrayView (and its mutable variant) is exposed as well. This one works only with linear tightly packed memory and thus is suitable for taking views onto bytes or bytearray, file contents and such. Both the strided and linear array views of course support the full Python slicing API. As an example, here’s how you can read an image in Python, pass its contents to a Magnum importer and get the raw pixel data back:
from magnum import trade def consume_pixels(pixels: np.ndarray): ... importer: trade.AbstractImporter = trade.ImporterManager().load_and_instantiate('AnyImageImporter') with open(filename, 'rb') as f: importer.open_data(f.readall()) image: trade.ImageData2D = importer.image2d(0) # green channel of a lower right quarter of a 256x256 image flipped upside down consume_pixels(image.pixels[128:128,::-1,1:2])
Just one question left — who owns the memory here, then? To answer that, let’s dive into Python’s reference counting.
Reference counting
In C++, views are one of the more dangerous containers, as they reference data owned by something else. There you’re expected to ensure the data owner is kept in scope for at least as long as the view on it. A similar thing is with other types — for example, a GL::Mesh may reference a bunch of GL::Buffers, or a Trade::AbstractImporter loaded from a plugin needs its plugin manager to be alive to keep the plugin library loaded.
The dim dashed lines show additional potential dependencies that would
happen with future zero-copy plugin implementations
— when the file format allows it, these would reference directly the
data in f
instead of storing a copy themselves.
However, imposing similar constraints on Python users would be daring too much, so all exposed Magnum types that refer to external data implement reference counting under the hood. The designated way of doing this with pybind11 is wrapping all your everything with std::shared_ptr. On the other hand, Magnum is free of any shared pointers by design, and adding them back just to make Python happy would make everyone else angry in exchange. What Magnum does instead is extending the so-called holder type in pybind11 (which doesn’t have to be std::shared_ptr; std::unique_ptr or a custom pointer types is fine as well) and storing references to instance dependencies inside it.
The straightforward way of doing this would be to take GL::Mesh,
subclass it into a PyMesh
, store buffer references inside it and then
expose PyMesh
as gl.Mesh instead. But compared to the holder type
approach this has a serious disadvantage where every API that works with
meshes would suddenly need to work with PyMesh
instead and that’s not
always possible.
For testing and debugging purposes, references to memory owners or other data are always exposed through the API — see for example ImageView2D.owner or gl.Mesh.buffers.
Zero-waste data slicing
One thing I got used to, especially when writing parsers, is to continually slice the input data view as the algorithm consumes its prefix. Consider the following Python code, vaguely resembling an OBJ parser:
view = containers.ArrayView(data) while view: # Comment, ignore until EOL if view[0] == '#': while view and view[0] != '\n': view = view[1:] # Vertex / face elif view[0] == 'v': view = self.parse_vertex(view) elif view[0] == 'f': view = self.parse_face(view) ...
On every operation, the view
gets some prefix chopped off. While not a
problem in C++, this would generate an impressively long reference chain in
Python, preserving all intermediate views from all loop iterations.
While the views are generally smaller than the data they refer to, with big
files it could easily happen that the overhead of views becomes larger than the
parsed file itself. To avoid such endless growth, slicing operations on views
always refer the original data owner, allowing the intermediate views to be
collected. In other words, for a containers.ArrayView.owner, view[:].owner is view.owner
always holds.
The less-than-great aspects of pybind11
Throwing C++ exceptions is actually really slow
While I was aware there’s some overhead involved with C++’s throw
, I
never guessed the overhead would be so big. In most cases, this would not be
a problem as exceptions are exceptional but there’s one little corner of Python
where you have to use them — iteration. In order to iterate anything,
Python calls __getitem__()
with an increasing index, and instead of
checking against __len__()
, simply waiting until it raises
IndexError. This is also how conversion from/to lists is used and also
how numpy.array() populates the array from a list-like type, unless the
type supports Buffer Protocol. Bindings for Magnum’s Vector3.__getitem__()
originally looked like this:
.def("__getitem__", [](const T& self, std::size_t i) -> typename T::Type { if(i >= T::Size) throw pybind11::index_error{}; return self[i]; })
Plain and simple and seemingly not a perf problem at all … until you start measuring:
This is further blown out of proportion in case of numpy.array() — looking at the sources of PyArray_FromAny(), it’s apparently hitting the out-of-bounds condition three times — first when checking for dimensions, second when calculating a common type for all elements and third when doing an actual copy of the data. This was most probably not worth optimizing assuming sane exception performance, however combined with pybind11, it leads to a massive slowdown:
As hinted by the plots above, there’s a few possible ways of countering the inefficiency:
A lot of overhead in pybind11 is related to exception translation which can be sidestepped by calling PyErr_SetString() and telling pybind11 an error is already set and it only needs to propagate it:
.def("__getitem__", [](const T& self, std::size_t i) -> typename T::Type { if(i >= T::Size) { PyErr_SetString(PyExc_IndexError, ""); throw pybind11::error_already_set{}; } return self[i]; })
As seen above, this results in a moderate improvement with exceptions taking ~1 µs less to throw (though for numpy.array() it doesn’t help much). This is what Magnum Bindings globally switched to after discovering the perf difference, and apart from PyErr_SetString(), there’s also PyErr_Format() able to stringify Python objects directly using
"%A"
— hard to beat that with any third-party solution.Even with the above, the
throw
and the whole exception bubbling inside pybind is still responsible for quite a lot, so the next step is to only call PyErr_SetString() and return nothing to pybind to indicate we want to raise an exception instead:.def("__getitem__", [](const T& self, std::size_t i) -> pybind11::object { if(i >= T::Size) { PyErr_SetString(PyExc_IndexError, ""); return pybind11::object{}; } return pybind11::cast(self[i]); })
This results in quite a significant improvement, reducing the exception overhead from about 3.5 µs to 0.4 µs. It however relies on a patch that’s not merged yet (see pybind/pybind11#1853) and it requires the bound API to return a typeless object instead of a concrete value as there’s no other way to express a “null” value otherwise.
- If there’s a possibility to use the Buffer Protocol, preferring it over
__getitem__()
. At first I was skeptical about this idea because the buffer protocol setup with pointers and shapes and formats and sizes and all related error checking certainly feels heavier than simply iterating a three-element array. But the relative heaviness of exceptions makes it a winner. Pybind has a builtin support, so why not use it. Well, except …
Let’s allocate a bunch of vectors and strings to do a zero-copy data transfer
Python’s Buffer Protocol, mentioned above, is a really nice approach for data transfers with minimal overhead — if used correctly. Let’s look again at the case of calling numpy.array() above:
It’s clear that converting a pure Python list to a numpy.array() is, even with all the exceptions involved, still faster than using pybind’s buffer protocol implementation to convert a Vector3 to it. In comparison, array.array() (which implements Buffer Protocol as well, only natively in plain C) is quite speedy, so there’s definitely something fishy in pybind11.
struct buffer_info { void *ptr; ssize_t itemsize; std::string format; ssize_t ndim; std::vector<ssize_t> shape; std::vector<ssize_t> strides; };
Oh, so that’s why.
The std::vector allocations (and std::string possibly as well, if
the format specifier is too long for small string optimization) in
pybind11::buffer_info
add up to the overhead, so I decided to sidestep
pybind11 altogether and interface directly with the underlying Python C API
instead. Because the Py_buffer structure is quite flexible, I
ended up pointing its members to statically defined data
for each matrix / vector type, making the buffer protocol operation completely
allocation-less. In case of containers.ArrayView and its strided
equivalent the structure points to their internal members, so nothing needs to
be allocated even in case of containers.StridedArrayView4D.
Additionally, operating directly with the C API allowed me to correctly
propagate readonly properties and the
above-mentioned data ownership as well.
Become a 10× programmer with this one weird trick
Compile times with pybind are something I can’t get used to at all. Maybe this
is nothing extraordinary when you do a lot of Modern C++, but an incremental
build of a single file taking 20 seconds is a bit too much for my taste. In
comparison, I can recompile the full Magnum (without tests) in 15 seconds. This
gets a lot worse when building Release, due to -flto
being passed to the
compiler — then an incremental build of that same file takes 90 seconds5,
large part of the time spent in Link-Time Optimization.
Fortunately, by another lucky accident, I recently discovered that GCC’s -flto
flag has a parallel option6 — so if you have 8 cores, -flto=8
will
make the LTO step run eight times faster, turning the above 90 seconds into
slightly-less-horrific 35 seconds. Imagine that. This has however a dangerous
consequence — the buildsystem is not aware of the LTO parallelism, so it’s
inevitable that it will happily schedule 8 parallelized link jobs at once,
bringing your machine to a grinding halt unless you have 32 GB RAM and most of
those free. If you use Ninja, it has job pools
where you can tell it to not fire up more than one such link job at once, but
as far as my understanding of this feature goes, this will not affect the
scheduling of compile and link in parallel.
Once Clang 9 is out (and once I get some free time), I want to unleash the new -ftime-trace option on the pybind code, to see if there’s any low-hanging fruit. But unfortunately in the long term I’m afraid I’ll need to replace even more parts of pybind to bring compile times back to sane bounds.
- 5.
- ^ To give a perspective, the cover image of this article (on the top) is generated from preprocessed output of the file that takes 90 seconds to build. About 1%, few faded lines in the front, is the actual bindings code. The rest — as far as your eyes can see — is STL and pybind11 headers.
- 6.
- ^ It’s currently opt-in, but GCC 10 is scheduled to have it enabled by default. If you are on Clang, it has ThinLTO, however I was not able to convince it to run parallel for me.
Everyone “just uses Sphinx”. You?
The obvious first choice when it comes to documenting Python code is to use Sphinx — everything including the standard library uses it and I don’t even remember seeing a single Python library that doesn’t. However, if you clicked on any of the above doc links, you probably realized that … no, Magnum is not using it.
Ever since the documentation search got introduced early last year, many developers quickly became addicted used to it. Whipping up some Sphinx docs, where both search performance and result relevance is extremely underwhelming, would be effectively undoing all usability progress Magnum made until now, so the only option was to bring the search to Python as well.
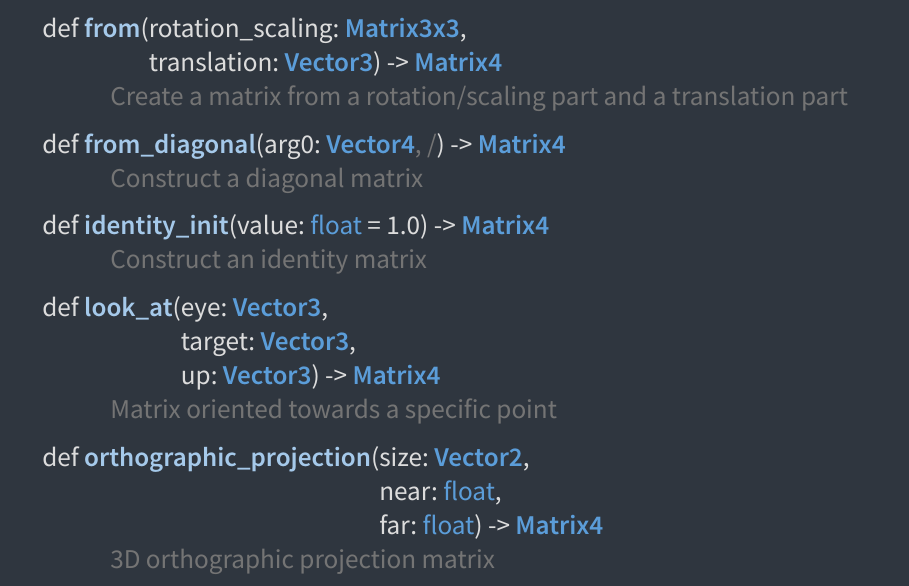
While at it, I made the doc generator aware of all kinds of type annotations, properly crosslinking everything to corresponding type definitions. And not just local types — similarly to Doxygen’s tagfiles, Sphinx has Intersphinx, so linking to 3rd party library docs (such as NumPy) or even the standard library is possible as well. Conversely, the m.css Python doc generator exports an Intersphinx inventory file, so no matter whether you use vanilla Sphinx or m.css, you can link to m.css-generated Python docs from your own documentation as well.
If you want to try it out on your project, head over to the m.css website for a brief introduction. Compared to Sphinx or Doxygen it behaves more like Doxygen, as it implicitly browses your module hierarchy and generates a dedicated page for each class and module. The way Sphinx does it was interesting for me at first, but over the time I realized it needs quite a lot of effort from developer side to organize well — and from the documentation reader side, it can lead to things being harder to find than they should be (for example, docs for str.splitlines() are buried somewhere in the middle of a kilometer-long page documenting all builtin types).
The doc generator resembles Sphinx, but I decided to experiment with a clean slate first instead of making it 100% compatible — some design decisions in Sphinx itself are historical (such as type annotations in the doc block instead of in the code itself) and it didn’t make sense for me to port those over. Well, at least for now, a full Sphinx compatibility is not completely out of question.
What’s next?
The work is far from being done — apart from exposing APIs that are not exposed yet (which is just a routine work, mostly), I’m still not quite satisfied with the performace of bound types, so on my roadmap is trying to expose a basic type using pure C Python APIs the most efficient way possible and then comparing how long does it take to instantiate that type and call methods on it. One of the things to try is a vectorcall call protocol that’s new in Python 3.8 (PEP590) and the research couldn’t be complete without also trying a similar thing in MicroPython.
~ ~ ~